Editor’s Note: Norman Katz, president of supply chain consultancy Katzscan Inc., writes a monthly column for Supply Chain Management Review. Katz’s column appears on the third Monday of each month.
A CIO Dive article published on Feb. 14 cited multiple surveys that reflected on project failures related to AI implementations.
According to the article:
- A Boston Consulting Group poll in 2023 discovered that two-thirds of C-suite leaders found their organization’s progress with generative AI was not up to par.
- An Everest Group survey in January 2024 revealed that CIOs find AI solutions difficult to scale even after successful pilots. The primary reasons are a lack of success metrics clarity (73%), concerns regarding costs (68%), and the speed at which the technology landscape rapidly changes (64%).
The article was supportive, though, as it explained that failures should not be looked at as dead ends but instead learning opportunities.
The article focused on the failures of generative AI projects. Granted, perhaps this was the intention of the article to only focus on generative AI. (This is really the only type of AI that we read and hear about.) My question is: what if the AI project failures were due to the selection of the wrong type of AI? What if the term “generative AI” has become a bit generic when referring to AI?
Generative AI creates new data from a given set of data. Examples of this are new text from existing text, or images from text prompts. Discriminative AI is used to differentiate between categories within a given set of data. An example of discriminative AI is assessing for manufacturing defects where the flaw is defined as being outside of the acceptable tolerance parameters. Discriminative AI is more typically used for fraud detection.
According to a Fast Company article of July 25, 2023, discriminative AI will lead generative AI in enterprise implementations because:
- Discriminative AI has superior accuracy and reliability than generative AI
- Discriminative AI is more robust to noise and outlier data than generative AI
- It is easier to understand how discriminative AI interprets data than generative AI
This is not to say that generative AI will not catch up to discriminative AI, nor is it to suggest that generative AI does not have a place in the business enterprise. What I am only saying here—as I have said in other articles on software—is that AI is a piece of software that is not just one thing: it is a technology tool to do a job. Selecting the right software, and at the right point of the business process, is the key to getting these (or any) software projects done and done correctly. The solution to a successful AI implementation, as noted at the end of the Fast Company article, maybe to place a discriminative AI product at the front or near the source of the data and let it differentiate the data within the defined categories, and then to have a generative AI product assess the parsed data after.
As we know from the number of interconnected systems, (e.g., ERP, EDI, automatic identification), that are needed to run our supply chains, the solution to a successful AI implementation is to first select the right software for the problem, and then acknowledge that a full end-to-end solution is likely to involve an integration of more than one system.
SC
MR


More Artificial Intelligence
- The hard job of teaching soft skills
- Nine questions are the key to AI success in building resilient supply chains
- AI is moving omnichannel closer to the customer
- Fighting Disruption: Synchronizing Supply Chain Execution
- Harnessing Edge Computing and AI Vision Systems for Real-Time Logistics Insights
- More Artificial Intelligence
What's Related in Artificial Intelligence

Explore
Topics
Procurement & Sourcing News
- Strengthening customer fulfillment: Building a strategic stakeholder network
- Trump picks former Wisconsin congressman Sean Duffy for DOT secretary
- Made in Mexico, manufactured by China
- Retail sales see gains in October, reports Commerce and NRF
- Geopolitical readiness in supply chains: Strategic challenges for leaders
- With capacity to spare, logistics real estate demand remains subdued
- More Procurement & Sourcing
Latest Procurement & Sourcing Resources
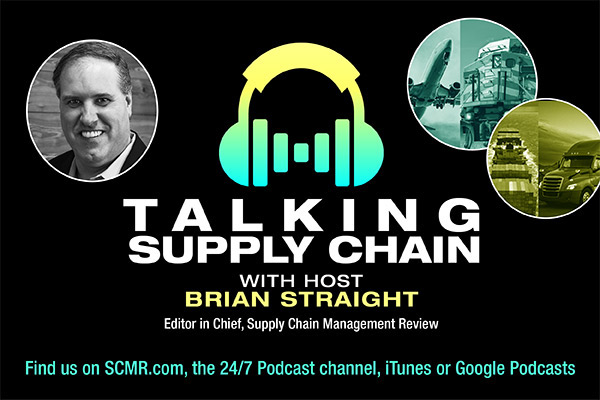
Subscribe

Supply Chain Management Review delivers the best industry content.
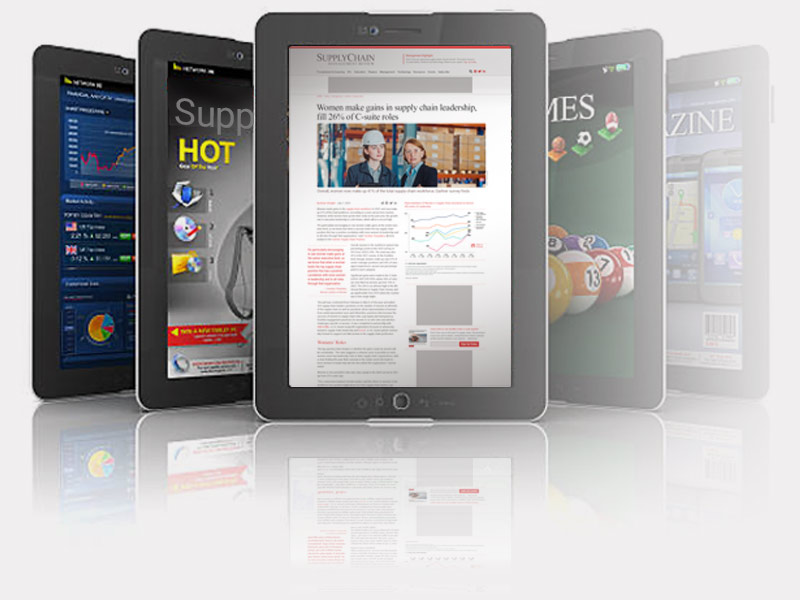
Editors’ Picks

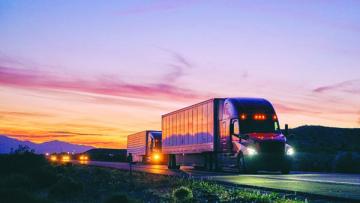

