Sorry, but your login has failed. Please recheck your login information and resubmit. If your subscription has expired, renew here.
January-February 2023
It feels like a normal holiday season. ”That was the report I got from the VP of global distribution at one of the country’s best known retail brands on the Monday before Black Friday. He added that the last time he felt that way was November of 2019. One of the perks of this job is that I get to talk to a lot of supply chain executives. In my conversations over the last year, I’ve learned two things. Operations, which was perhaps hardest hit at the start of the pandemic, has learned to operate in this new environment. Sure, there are still hiccups caused by absenteeism and inventory shortages. But those are situational—give them supply and… Browse this issue archive.Need Help? Contact customer service 847-559-7581 More options
Companies recruit specialists to help them generate, manage and interpret the flood of data that is the lifeblood of modern supply chains. Hence, job titles such as data analyst, data engineer and data scientist are relatively recent additions to the supply chain management discipline. How are practitioners harnessing these talents to innovate and improve supply chain performance?
A roundtable organized by the MIT Center for Transportation & Logistics and hosted by home goods retailer Wayfair explored the ROI of supply chain data. Various topics were discussed by the visiting supply chain practitioners from corporate members of the MIT Supply Chain Exchange. But three topics stood out as particularly challenging: how to define these specialist roles, how to integrate them into supply chain teams and how to manage their relationships with other stakeholders.
Confusion over role definitions
Data analytics, engineering and science involve different areas of expertise, yet there was some confusion at the roundtable over how they are differentiated within the supply chain domain.
One roundtable attendee associated data engineering with writing code and software automation. He perceived data science as an operations research type of role oriented toward applications such as the use of machine learning in demand forecasting. Another practitioner saw data engineers as responsible for identifying the right data sources and, increasingly, for supporting data governance. One company said it employed data engineers to capture and format existing data for applications like data visualization. There were many different perspectives.
Even the specialists themselves are not always clear on how the different roles are defined. “Everyone seems to think they are a data scientist when they are not,” commented a practitioner. He was informed that the company employed about 50 data scientists but discovered that there were three to four individuals who actually performed this role while the rest were basic spreadsheet analysts. Moreover, “recruiters may not know the right questions to ask” or have a clear idea of how specific data management skills profiles fit specific technical roles.
A leading manufacturer has added “business translator” to the mix of data management roles. In addition to being technically proficient, this individual is well versed in the business implications of data management. He or she translates the work of technical experts into a language senior management can understand, while ensuring that this work remains aligned with the company’s business objectives. The position can be filled by a “business-facing analytics leader” according to the roundtable participant.
Team-building issues
Melding these skills together into a team is not easy. “How do you differentiate analysts from data scientists, by background? And what problems do you want them to solve?” an attendee asked. Also: “The business translator is our highest-level resource, but where should they sit in the team?”
One practitioner explained his organization starts by hiring data scientists when creating a data team. His reasoning: “Because they can give you the ROI you need to gain buy-in from executives and IT.” Next, it hires data engineers “to improve the data flow and quality.” He described the data engineer and science disciplines as “salt and pepper” in that they are different but ought to be deployed together. Other attendees expressed a preference for hiring business translators and data analysts first, reflecting the participants’ different core needs. In general, the work undertaken by data scientists should be actionable and have the potential to be leveraged and scaled, it was suggested.
Attracting sought-after data experts to the supply chain field is a perennial problem. However, today’s climate of uncertainty is a boon for hiring managers because data experts are keen to apply their expertise to complex, global problems that have a human impact. Supply chain data roles check all these boxes. “Supply chain challenges involve lots of variables and you’re often dealing with the unknown,” observed one participant. It doesn’t hurt that awareness of the supply chain function’s criticality has increased.
An attendee recounted a hiring story that illustrates this effect. The company was interviewing a banker for a data job. The individual was asked how often his bank changes code, and he answered maybe twice per year. When the candidate learned that the company’s practice is to change code perhaps 10 times per day, he immediately wanted the job.
Still, supply chain’s notoriety only goes so far as an incentive to join the profession. Data experts value working in teams on challenging data-related problems first; interest in the supply chain domain comes second, pointed out an attendee.
Moreover, the likelihood of retaining new recruits often depends on individual lifestyle choices, and whether the job meets a person’s early expectations. An attendee said that for her company making the retail business attractive for “someone in their mid-twenties who wants do to something more challenging” can be a steep uphill battle. “The culture is a big factor,” she noted, “we spend a lot of time trying to develop a data-driven culture, integrating data experts in the business processes to elevate their visibility and impact.”
A modern-day challenge is how to develop teams of supply chain practitioners and data specialists that work remotely. “We find that getting everyone in the same room is more effective, but this can be an ongoing struggle,” said a participant.
Developing external links
A key capability that companies look for when hiring data specialists is an ability to collaborate. “Communication [skills] comes when they join a team but getting to a problem statement can be tough and this needs a lot of collaboration” commented one practitioner.
Collaborating and communicating with parties outside of the team—especially business leaders—can be a notable challenge for data engineers and scientists. The presence of a business translator smooths the way, but may not be a complete solution.
For example, an external leader might hesitate to partner with technical specialists “because they [leaders] are not comfortable in their domain and may not be able to answer their [the specialist’s] questions,” said a participant. In other words, a senior manager’s lack of knowledge in a particular area may become exposed, and this fear becomes an obstacle in projects that require close cooperation with technical experts. No one wants to lay themselves open to embarrassment, so some managers shun such collaborative relationships.
This is particularly troublesome when the issues involved are data related. “When the rubber hits the road and it is your intuition versus the data, your credibility is the key,” said the roundtable attendee. Further, “when things don’t align with the data, they [non-technical managers] might question the data.” A possible solution is to “put data people in the field” he suggested to give them a deeper appreciation of these issues.
A relational pain point that was a recurrent theme at the roundtable is a lack of communication between the new generation of data managers and IT departments.
In many ways IT represents the original data management resource within companies. Yet, “it can be a struggle to get IT to work with us,” said a participant “We start a project that requires IT support, and a month becomes two-and-a-half years.” IT is often not agile enough to tackle the many data-related problems that have become central to the task of improving supply chain efficiency, especially when it comes to building multiple models. One company has established special teams to quickly build models when other internal resources are unable to meet such needs.
A practitioner attendee was given responsibility for improving a data analytics team that was set up around two years previously. “When I joined it was a mess,” she said. The teams’ relationship with IT was tarnished, creating multiple data quality issues on a daily basis. She halted new development work and focused on key problem areas such as how to work better with IT personnel.
Data teams will unlock more value
Data specialists are more important than ever given the growing volumes of data available to companies and the increasing sophistication of related management tools. They play a critical role in helping supply chain teams to manage uncertainty, or “how we see around corners” to anticipate disruptions, said a roundtable attendee.
However, integrating these specialists into operational teams and leveraging their skills to support decision-making is still a work in progress.
Other challenges that need to be addressed include managing external contractors in data management and tackling data governance issues.
The role of visualization is another challenge that warrants special attention. For example, sophisticated visualization techniques that present data in easily digestible formats can reduce the need for analysts to interpret the data. The technology can also provide a platform for inter-disciplinary collaboration. MIT CTL’s Computational and Visual Education (CAVE) Lab* is using the technology to enable different disciplines including supply chain, finance, and marketing to analyze the competitive implications of supply chain design changes.
An attendee suggested that as data management within supply chains matures, teams’ perspectives shift closer to the customer end of the business. Mature teams find ways to use their combined talents to unlock value by enhancing the customer experience.
Along the way, they are learning some important lessons. For example, an attendee observed that in his company’s experience, a higher ROI comes from adding data to a model rather than attempting to use a better algorithm.
Insights like these will surely enhance the value of data management going forward.
SC
MR
Sorry, but your login has failed. Please recheck your login information and resubmit. If your subscription has expired, renew here.
January-February 2023
It feels like a normal holiday season. ”That was the report I got from the VP of global distribution at one of the country’s best known retail brands on the Monday before Black Friday. He added that the last time… Browse this issue archive. Access your online digital edition. Download a PDF file of the January-February 2023 issue.Companies recruit specialists to help them generate, manage and interpret the flood of data that is the lifeblood of modern supply chains. Hence, job titles such as data analyst, data engineer and data scientist are relatively recent additions to the supply chain management discipline. How are practitioners harnessing these talents to innovate and improve supply chain performance?
A roundtable organized by the MIT Center for Transportation & Logistics and hosted by home goods retailer Wayfair explored the ROI of supply chain data. Various topics were discussed by the visiting supply chain practitioners from corporate members of the MIT Supply Chain Exchange. But three topics stood out as particularly challenging: how to define these specialist roles, how to integrate them into supply chain teams and how to manage their relationships with other stakeholders.
Confusion over role definitions
Data analytics, engineering and science involve different areas of expertise, yet there was some confusion at the roundtable over how they are differentiated within the supply chain domain.
One roundtable attendee associated data engineering with writing code and software automation. He perceived data science as an operations research type of role oriented toward applications such as the use of machine learning in demand forecasting. Another practitioner saw data engineers as responsible for identifying the right data sources and, increasingly, for supporting data governance. One company said it employed data engineers to capture and format existing data for applications like data visualization. There were many different perspectives.
Even the specialists themselves are not always clear on how the different roles are defined. “Everyone seems to think they are a data scientist when they are not,” commented a practitioner. He was informed that the company employed about 50 data scientists but discovered that there were three to four individuals who actually performed this role while the rest were basic spreadsheet analysts. Moreover, “recruiters may not know the right questions to ask” or have a clear idea of how specific data management skills profiles fit specific technical roles.
A leading manufacturer has added “business translator” to the mix of data management roles. In addition to being technically proficient, this individual is well versed in the business implications of data management. He or she translates the work of technical experts into a language senior management can understand, while ensuring that this work remains aligned with the company’s business objectives. The position can be filled by a “business-facing analytics leader” according to the roundtable participant.
Team-building issues
Melding these skills together into a team is not easy. “How do you differentiate analysts from data scientists, by background? And what problems do you want them to solve?” an attendee asked. Also: “The business translator is our highest-level resource, but where should they sit in the team?”
One practitioner explained his organization starts by hiring data scientists when creating a data team. His reasoning: “Because they can give you the ROI you need to gain buy-in from executives and IT.” Next, it hires data engineers “to improve the data flow and quality.” He described the data engineer and science disciplines as “salt and pepper” in that they are different but ought to be deployed together. Other attendees expressed a preference for hiring business translators and data analysts first, reflecting the participants’ different core needs. In general, the work undertaken by data scientists should be actionable and have the potential to be leveraged and scaled, it was suggested.
Attracting sought-after data experts to the supply chain field is a perennial problem. However, today’s climate of uncertainty is a boon for hiring managers because data experts are keen to apply their expertise to complex, global problems that have a human impact. Supply chain data roles check all these boxes. “Supply chain challenges involve lots of variables and you’re often dealing with the unknown,” observed one participant. It doesn’t hurt that awareness of the supply chain function’s criticality has increased.
An attendee recounted a hiring story that illustrates this effect. The company was interviewing a banker for a data job. The individual was asked how often his bank changes code, and he answered maybe twice per year. When the candidate learned that the company’s practice is to change code perhaps 10 times per day, he immediately wanted the job.
Still, supply chain’s notoriety only goes so far as an incentive to join the profession. Data experts value working in teams on challenging data-related problems first; interest in the supply chain domain comes second, pointed out an attendee.
Moreover, the likelihood of retaining new recruits often depends on individual lifestyle choices, and whether the job meets a person’s early expectations. An attendee said that for her company making the retail business attractive for “someone in their mid-twenties who wants do to something more challenging” can be a steep uphill battle. “The culture is a big factor,” she noted, “we spend a lot of time trying to develop a data-driven culture, integrating data experts in the business processes to elevate their visibility and impact.”
A modern-day challenge is how to develop teams of supply chain practitioners and data specialists that work remotely. “We find that getting everyone in the same room is more effective, but this can be an ongoing struggle,” said a participant.
Developing external links
A key capability that companies look for when hiring data specialists is an ability to collaborate. “Communication [skills] comes when they join a team but getting to a problem statement can be tough and this needs a lot of collaboration” commented one practitioner.
Collaborating and communicating with parties outside of the team—especially business leaders—can be a notable challenge for data engineers and scientists. The presence of a business translator smooths the way, but may not be a complete solution.
For example, an external leader might hesitate to partner with technical specialists “because they [leaders] are not comfortable in their domain and may not be able to answer their [the specialist’s] questions,” said a participant. In other words, a senior manager’s lack of knowledge in a particular area may become exposed, and this fear becomes an obstacle in projects that require close cooperation with technical experts. No one wants to lay themselves open to embarrassment, so some managers shun such collaborative relationships.
This is particularly troublesome when the issues involved are data related. “When the rubber hits the road and it is your intuition versus the data, your credibility is the key,” said the roundtable attendee. Further, “when things don’t align with the data, they [non-technical managers] might question the data.” A possible solution is to “put data people in the field” he suggested to give them a deeper appreciation of these issues.
A relational pain point that was a recurrent theme at the roundtable is a lack of communication between the new generation of data managers and IT departments.
In many ways IT represents the original data management resource within companies. Yet, “it can be a struggle to get IT to work with us,” said a participant “We start a project that requires IT support, and a month becomes two-and-a-half years.” IT is often not agile enough to tackle the many data-related problems that have become central to the task of improving supply chain efficiency, especially when it comes to building multiple models. One company has established special teams to quickly build models when other internal resources are unable to meet such needs.
A practitioner attendee was given responsibility for improving a data analytics team that was set up around two years previously. “When I joined it was a mess,” she said. The teams’ relationship with IT was tarnished, creating multiple data quality issues on a daily basis. She halted new development work and focused on key problem areas such as how to work better with IT personnel.
Data teams will unlock more value
Data specialists are more important than ever given the growing volumes of data available to companies and the increasing sophistication of related management tools. They play a critical role in helping supply chain teams to manage uncertainty, or “how we see around corners” to anticipate disruptions, said a roundtable attendee.
However, integrating these specialists into operational teams and leveraging their skills to support decision-making is still a work in progress.
Other challenges that need to be addressed include managing external contractors in data management and tackling data governance issues.
The role of visualization is another challenge that warrants special attention. For example, sophisticated visualization techniques that present data in easily digestible formats can reduce the need for analysts to interpret the data. The technology can also provide a platform for inter-disciplinary collaboration. MIT CTL’s Computational and Visual Education (CAVE) Lab* is using the technology to enable different disciplines including supply chain, finance, and marketing to analyze the competitive implications of supply chain design changes.
An attendee suggested that as data management within supply chains matures, teams’ perspectives shift closer to the customer end of the business. Mature teams find ways to use their combined talents to unlock value by enhancing the customer experience.
Along the way, they are learning some important lessons. For example, an attendee observed that in his company’s experience, a higher ROI comes from adding data to a model rather than attempting to use a better algorithm.
Insights like these will surely enhance the value of data management going forward.
SC
MR
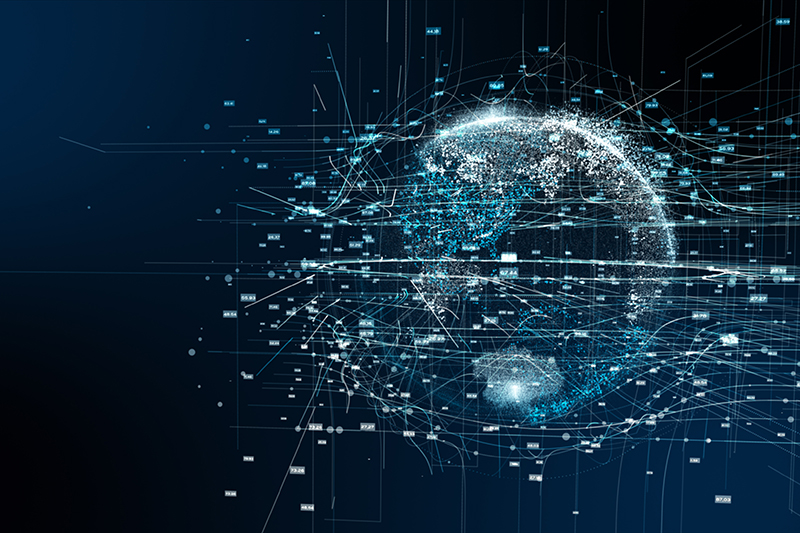

Latest Supply Chain News
- Retail sales see gains in October, reports Commerce and NRF
- Balancing green and speed: Home delivery insights from the pandemic era
- AdventHealth named top healthcare supply chain by Gartner
- Geopolitical readiness in supply chains: Strategic challenges for leaders
- Unlocking retention: The role employee engagement plays
- More News
Latest Podcast
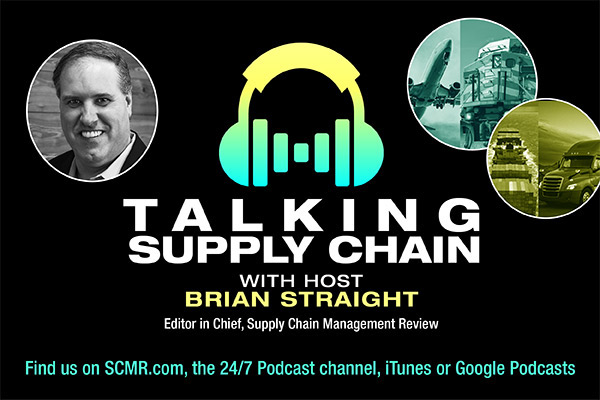
Explore
Business Management News
- Retail sales see gains in October, reports Commerce and NRF
- Balancing green and speed: Home delivery insights from the pandemic era
- AdventHealth named top healthcare supply chain by Gartner
- Unlocking retention: The role employee engagement plays
- Can supply chain managers embrace an entrepreneurial mindset?
- Challenges to ESG reporting
- More Business Management
Latest Business Management Resources
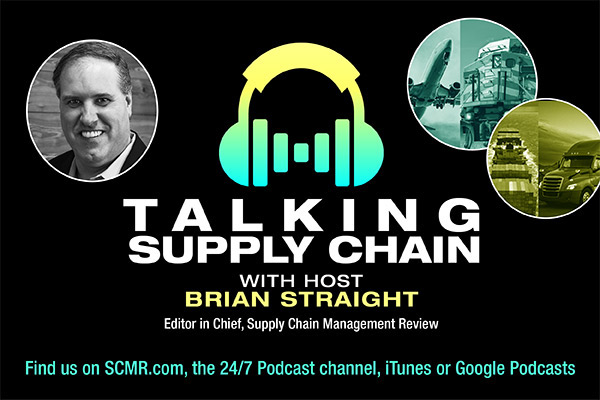
Subscribe

Supply Chain Management Review delivers the best industry content.
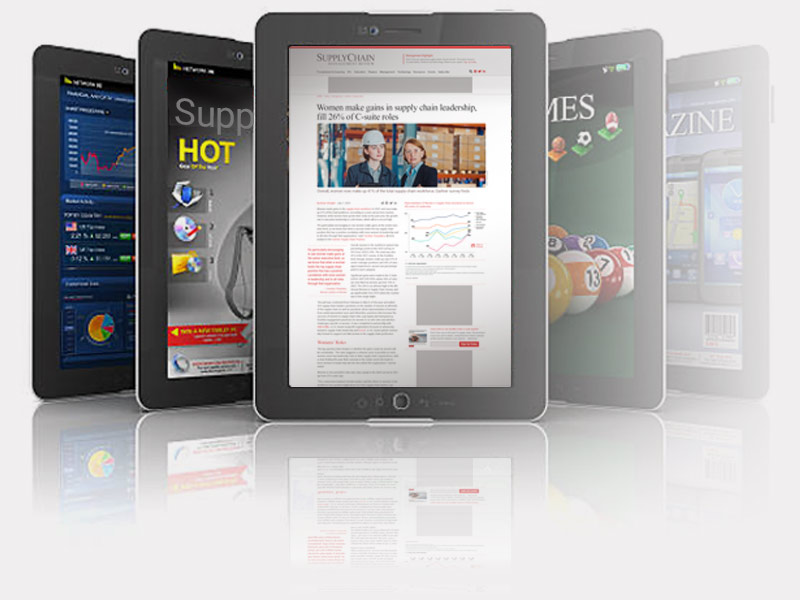
Editors’ Picks

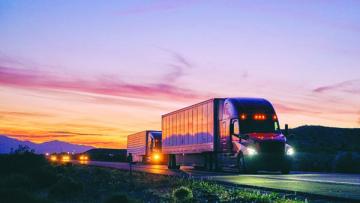

