Supply chain project management has evolved, shifting from a focus on efficiency to navigating a complex landscape influenced by globalization, technology, and changing consumer preferences. The vulnerabilities exposed during events like the COVID-19 pandemic underscore the need for adaptability. The pandemic posed challenges, disrupting production, leading to closures, and causing delays and increased costs in global transportation.
Additionally, unpredictable shifts in consumer behavior created demand fluctuations, impacting industries differently. Inventory management became more challenging, resulting in shortages or excess inventory. Supplier reliability and labor shortages further strained production capacity.
The crisis highlighted the necessity for digital transformation, remote work, and technology adoption in supply chain management. Regulatory changes and economic downturns added complexity to cross-border supply chains. Financial strain emphasized the importance of robust risk management, leading to a renewed focus on building resilient and agile supply chains. Businesses now invest in technology, diversify suppliers, and reassess inventory strategies.
Intelligent software enhances decision-making and risk management, facilitating collaboration throughout the supply chain. For instance, during sudden demand changes due to lockdowns, the software swiftly analyzes data, enabling real-time adjustments to inventory, production, and distribution. This adaptability ensures a responsive and agile supply chain, surpassing traditional approaches for efficiency and customer satisfaction.
The promise of intelligent software
In the current technological realm, intelligent software signifies more than just automation; it melds advanced algorithms, artificial intelligence, and machine learning to emulate human cognitive abilities. Unlike its conventional counterparts, this software learns, adapts, and autonomously recommends actions, excelling in data analysis and trend prediction. Its continuous adaptation based on feedback refines its performance over time.
Machine learning (ML) provides specialized models for supply chain management. For demand forecasting, ARIMA and regression models use historical data and additional factors like pricing to predict demand. Inventory optimization employs algorithms such as “random forest” for categorization, ensuring efficient stock levels. Supplier evaluation uses cluster analysis based on cost and reliability metrics. Transportation optimization employs simulated annealing for routing efficiency. Warehouse management leverages ML for anomaly detection and optimization of picking paths. In risk management, survival analysis identifies supply chain vulnerabilities for proactive planning. Customer segmentation techniques offer targeted strategies, boosting satisfaction. In essence, integrating these ML models enhances efficiency, resilience, and value across the supply chain.
Beyond operational improvements, intelligent software reshapes traditional supply chain challenges:
• Predictive analysis and forecasting. Moving beyond reliance on historical data, intelligent software uses real-time insights and market trends for precise demand forecasts, aiding in risk mitigation and inventory optimization.
• Optimized decision-making: Amidst complex supply chain dynamics, the software synthesizes data to aid decision-makers, ensuring more strategic choices.
• Enhanced operational efficiency: By integrating systems and automating tasks, it promotes collaboration and agility, ensuring streamlined operations and customer satisfaction.
• Resilience and risk management: In a volatile global landscape, the software evaluates risks, simulates scenarios, and devises strategies to bolster resilience and counteract disruptions.
How intelligent software could make a difference in specific situations
- Demand volatility amidst global events. The COVID-19 pandemic triggered significant demand shifts, straining supply chains. Intelligent software, with real-time analytics, could have monitored consumer behaviors, identified disruptions, and gauged inventory levels. Such insights would have refined demand forecasts, allowing organizations to adjust production and prioritize shipments, mitigating stockout risks and excess inventory costs.
- Supply chain disruptions due to geopolitical tensions. Geopolitical uncertainties can disrupt supply chains. Intelligent software could pre-emptively identify vulnerabilities, highlighting dependencies on specific regions or suppliers. Through simulations and alternative sourcing evaluations, it would have enabled organizations to devise resilient strategies, ensuring uninterrupted operations amid external disruptions.
- Quality control and recall management. Product recalls pose financial and reputational risks. Intelligent software, with advanced analytics, monitors production for deviations from quality standards. Using predictive analytics, it could anticipate issues, facilitating timely interventions, minimizing recall extents, and preserving brand reputation.
- Transportation and logistics optimization. Efficient transportation is crucial for supply chain success. Intelligent software, leveraging predictive analytics, would analyze factors like traffic and weather to optimize transportation strategies. This would reduce delays, enhance resource use, and boost supply chain effectiveness.
- Inventory management in seasonal industries. Seasonal industries face inventory challenges due to fluctuating demand and product perishability. Intelligent software, utilizing machine learning, analyzes sales trends and market dynamics to offer precise demand forecasts and inventory recommendations. This ensures optimal inventory levels, reduces holding costs, and capitalizes on market opportunities.
Conclusion
The evolving landscape of supply chain management highlights the transformative potential of integrating intelligent software. This technology offers benefits such as improved decision-making, streamlined operations, and proactive risk mitigation, with the potential to enhance customer satisfaction. However, caution is advised due to challenges like implementation complexities and data security risks. Emphasizing the need for a phased adoption strategy, starting with pilot projects, and fostering collaboration among diverse teams and stakeholders is crucial. Concurrent investment in talent development is underscored to equip the workforce with the necessary skills. In conclusion, a balanced, strategic, and human-centric approach to integrating intelligent software is key for operational excellence.
About the author:
Ahana Roy Choudhury is a senior software developer for Dassault Systemes. Choudhury builds business intelligence applications and tools, particularly focusing on web-based project management and other tracking solutions that facilitate precise tracking and optimization of crucial business metrics, featuring a sophisticated analytics module.
SC
MR
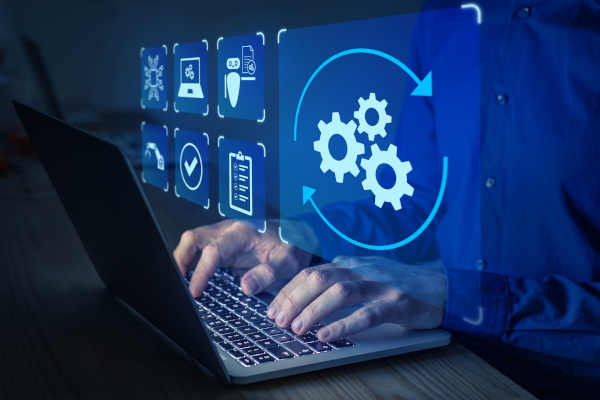

More Inventory Management
- How to improve demand forecasts for new product families
- Cross-border transport 2024: Navigating the surge
- Looking back at NextGen 2024
- How to make your CFO a supply chain superfan
- AI is moving omnichannel closer to the customer
- E-tailing update: Brick-and-mortar retailers struck back
- More Inventory Management
Latest Resources

Explore
Topics
Procurement & Sourcing News
- How to improve demand forecasts for new product families
- Aggregators sitting on the throne of Africa’s e-commerce supply chains: What lessons can we learn?
- Cross-border transport 2024: Navigating the surge
- Benchmarking the complexity of ESG reporting
- Looking back at NextGen 2024
- The Corporate Sustainability Due Diligence Directive
- More Procurement & Sourcing
Latest Procurement & Sourcing Resources
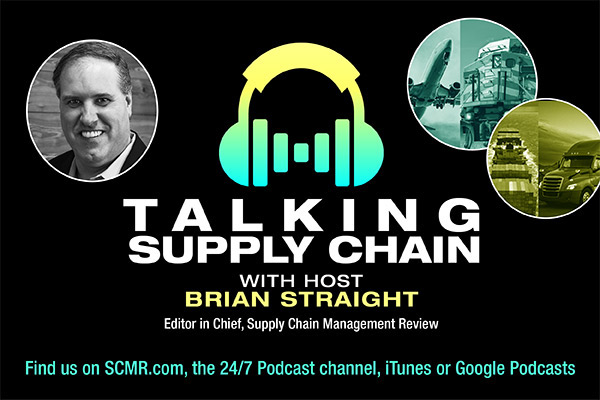
Subscribe

Supply Chain Management Review delivers the best industry content.
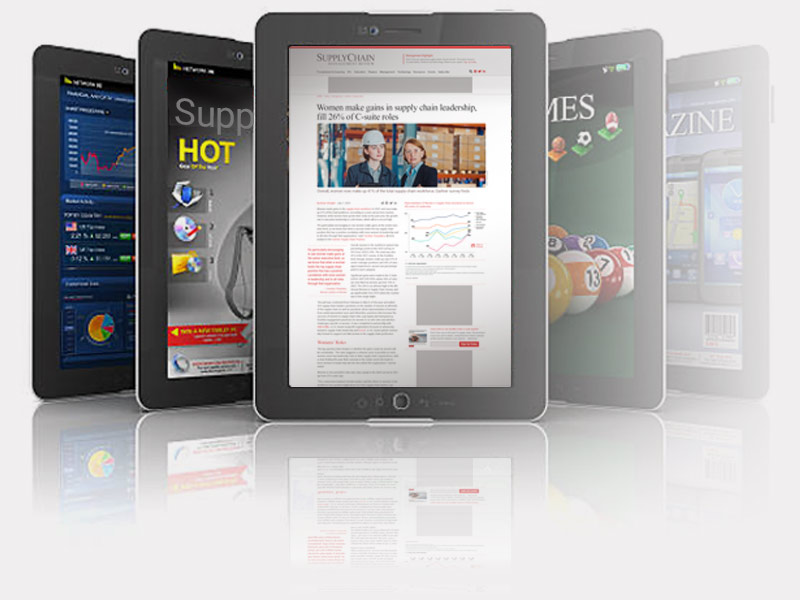
Editors’ Picks



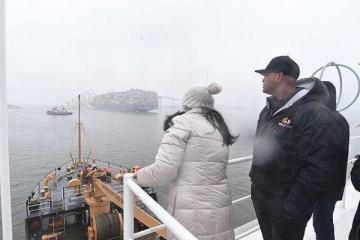